Compare performance and cost impact of switching LLMs
nOps AI Model Provider Recommendations help GenAI teams cut LLM spend by switching to lower-cost providers — like replacing OpenAI models with Claude or Nova tiers on AWS Bedrock — to reduce costs by up to 90% while maintaining similar performance.
Starting today, every recommendation includes a Quality Score based on the industry-standard MMLU benchmark, so you can evaluate accuracy impact as well as cost savings before making a switch.
In the example below, a conversational service running GPT-4o costs $112,456 per month. Our engine flags that the same prompt mix fits Nova Pro on Bedrock, saving approximately $98,047 per month (87.0% savings) while performing similarly or even better (up to 50% increase in quality) in some cases.
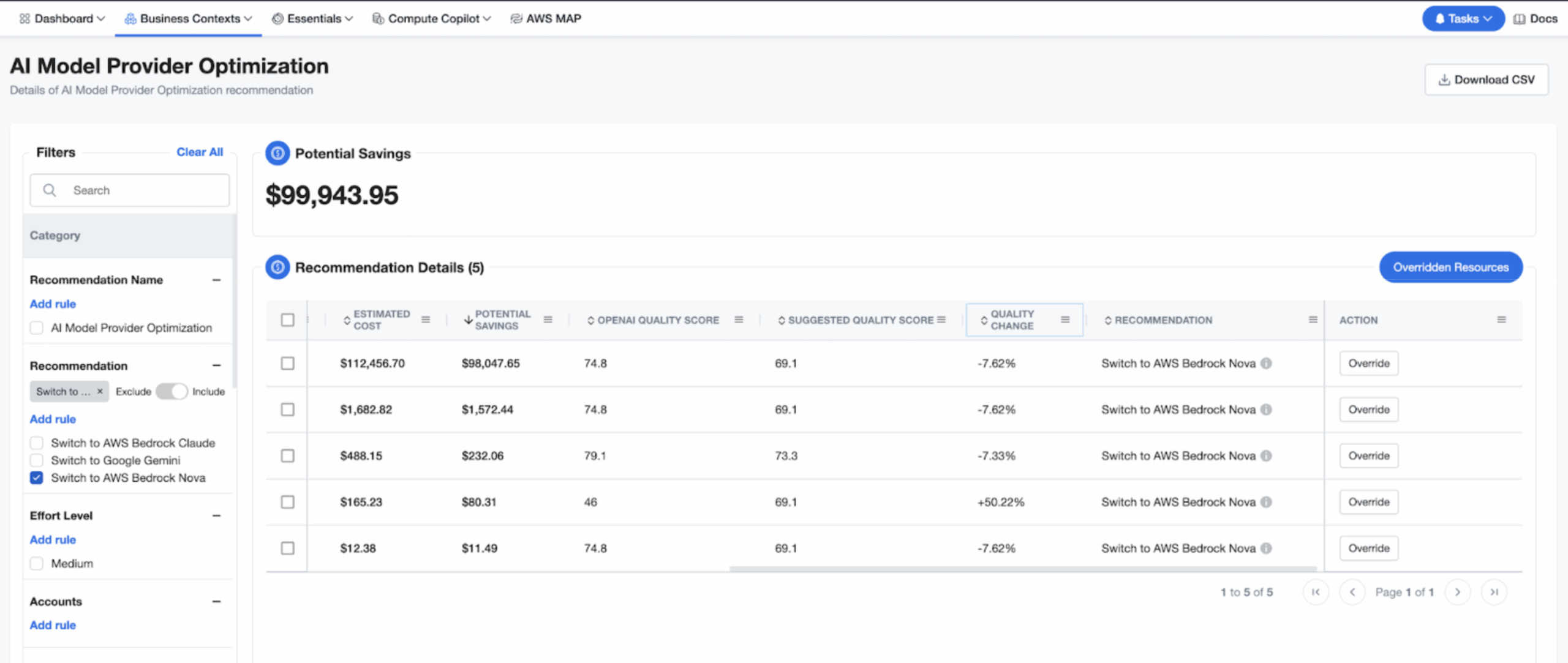
Quality Score
The quality score is based on the MMLU (Massive Multitask Language Understanding) benchmark, which reflects how well an LLM performs across 57 general-purpose tasks.
How to Get Started
Quality Change score: Alongside projected dollar savings, the dashboard now shows Quality Change (%). A positive number means the recommended model is more accurate; a small negative (≤ -15%) typically has minimal impact.
Detailed explanations: Find out the impact of the proposed switch (e.g., GPT-4o → AWS Bedrock Claude): pricing, capability notes, Quality Change score, and the exact monthly savings you can expect.
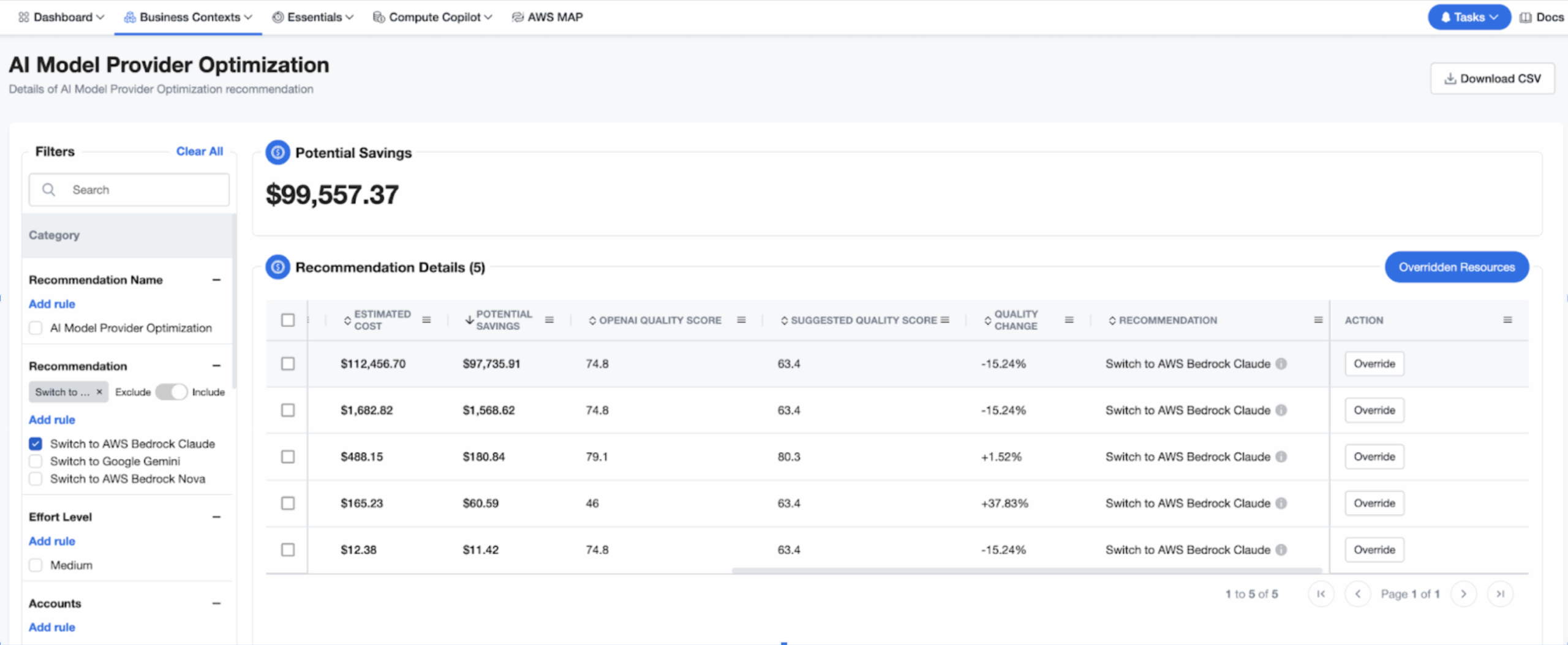
Does a 5-15% Quality Drop Matter?For most conversational and summarisation workloads, a ≤ 15-percent dip on MMLU typically has no noticeable effect on output — translating to minor phrasing differences, not factual errors. Studies show that users don’t perceive changes until the LLM quality metric drops ~20%. In other words, you can often realize double-digit savings with no visible impact to end users. |
How to Get Started
To access the updated recommendations, log in to nOps and navigate to the AI Model Provider Recommendations dashboard in nOps Cost Optimization.
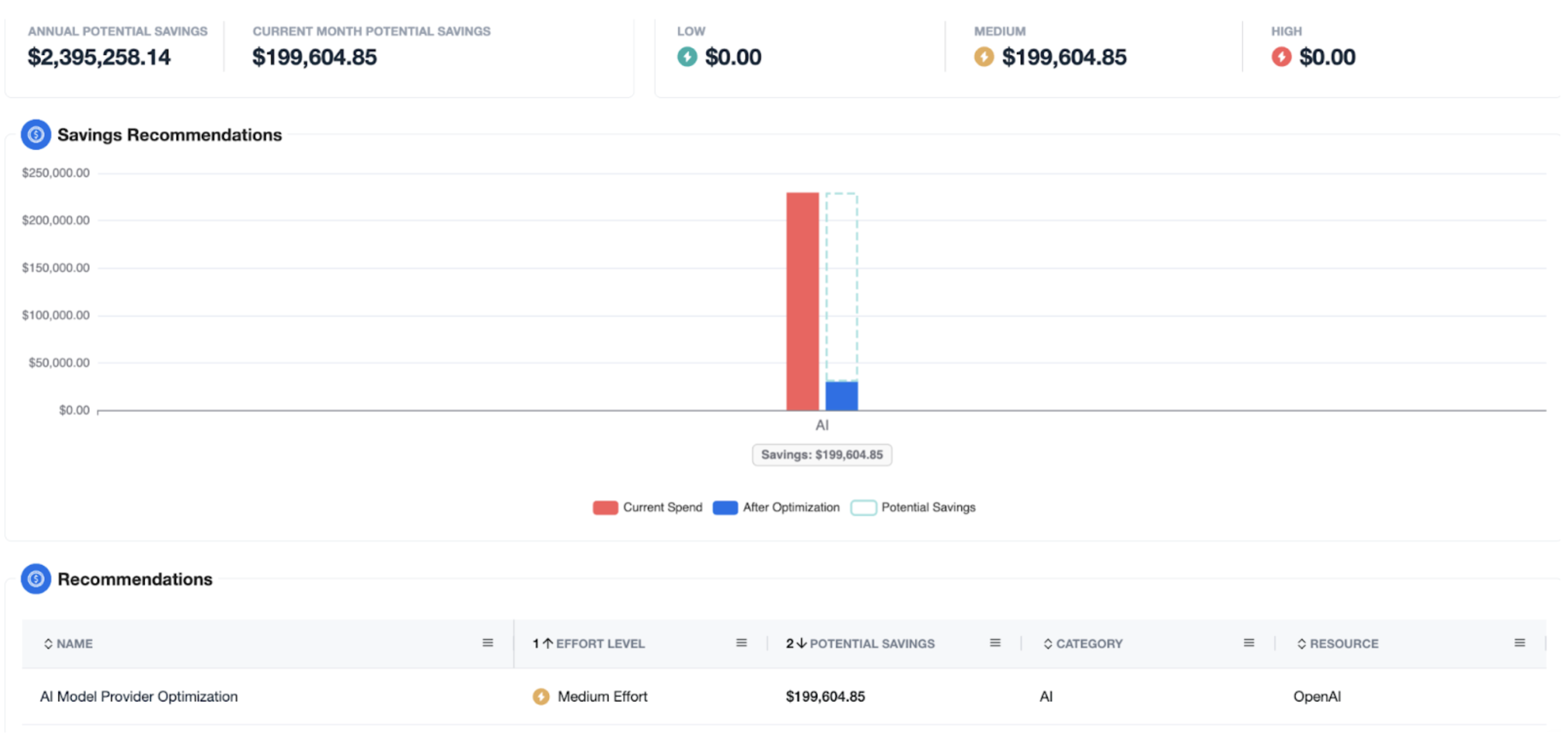
If you're already on nOps...
Have questions about AI Model Provider Recommendations? Need help getting started? Our dedicated support team is here for you. Simply reach out to your Customer Success Manager or visit our Help Center. If you’re not sure who your CSM is, send our Support Team an email.
If you’re new to nOps…
Ranked #1 on G2 for cloud cost management and trusted to optimise $2B+ in annual spend, nOps gives you automated GenAI savings with complete confidence. Book a demo to start saving on LLM cost without compromising on performance.